The SLF maintains an operational snow-hydrological service (OSHD) that continuously provides analyses and forecasts of the spatial and temporal distribution of snow water resources in Switzerland, facilitating improved hydrological forecasting and timely regulation of lake and reservoir levels.
In Switzerland, snow plays a major role in the water cycle. The snowmelt influences the seasonal runoff dynamics and can – often in combination with other factors – give rise to flooding or droughts. Since 2009 we have therefore been hosting an operational snow-hydrological service which continuously analyses the spatial and temporal distribution of snow in Switzerland. Today, OSHD services contribute to national and cantonal hydrological forecasting, natural hazard mitigation, and provide data for partner services such as the weather forecast by MeteoSwiss and our in-house avalanche warning.
Operational analyses
The OSHD produces daily snow-hydrological analyses from November to June containing a variety of information. The OSHD uses a physics-based modeling chain to predict snowmelt runoff, monitor snow water resources, and other variables of interest. Maps showing snow depth (A), snow water equivalent (B), runoff from snow melt (C), and state of the snow cover (D) are core model results that depict the snow situation (Figure 1). These maps are especially helpful for forecasting floods that arise during heavy rain-on-snow events.
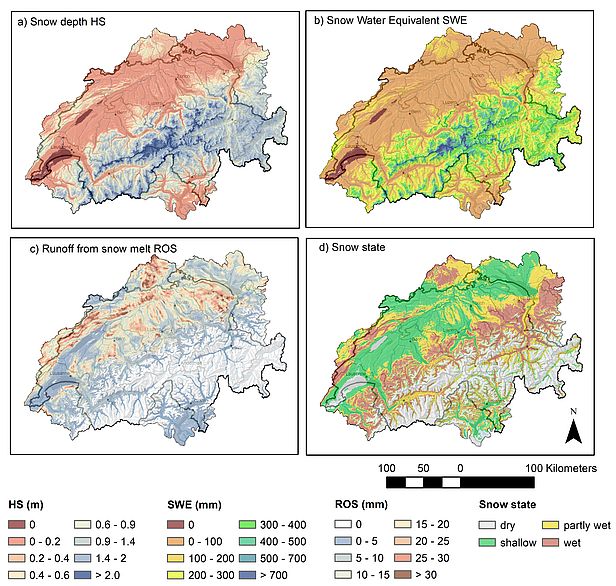
Snow water equivalent climatologies, in turn, reflect the temporal evolution of the current snow water reservoir and allow comparisons to be made with past winters (Figure 2). The winter 2021/22, e.g., showed unusually low snow amounts which had important implications for the regulation of lake and reservoir levels during the snowmelt period. The products displayed in Figure 1 and 2 are of high relevance for hydrological analysis. The OSHD is integrated into the forecasting and warning activities of the responsible national agencies. In the field of snow hydrology the Federal Office for the Environment (FOEN), the Federal Office of Meteorology and Climatology (MeteoSwiss), and the WSL Institute for Snow and Avalanche Research SLF work closely together. Weekly analysis by the OSHD are integrated into the Hydrological bulletin published by FOEN. Furthermore, the snow reserve is also important for the Swiss hydropower sector, and our data is published on the Energy Dashboard Switzerland by the Swiss Federal Office of Energy (SFOE).

The OSHD also produces new snow forecasts with varying lead-times (Figure 3). Together with total snow depth maps (Figure 1A), these products form a bases for the avalanche forecasting service operated by the SLF for assessing the current avalanche danger. Both the total snow depth and new snow depth products are published on White Risk and MeteoSwiss.
Methods
The OSHD model runs undergo updates eight times daily, utilizing a state-of-the-art model system designed to provide accurate analysis and forecasts of snow cover dynamics across Switzerland. The OSHD system comprises a set of advanced snow hydrological models, precisely representing snow conditions in low-land, forested, and high-alpine regions of Switzerland. To ensure precision, the model integrates station data, high-resolution meteorological forcing data, and various reanalysis products, employing cutting-edge data assimilation and meteorological downscaling methods. The OSHD system offers a range of models, each tailored to specific applications such as flood forecasting and climatological analysis.
Please refer to the publication "Operational Snow-Hydrological Modeling for Switzerland" for more in-depth insights into our model system. This article provides comprehensive information on our snow modeling system's technical aspects and methodologies.
Contact
Links
Publications
Mott, R., Winstral, A., Cluzet, B., Helbig, N., Magnusson, J., Mazzotti, G., Quéno, L., Schirmer, M., Webster, C., & Jonas, T. (2023). Operational snow-hydrological modeling for Switzerland. Frontiers in Earth Science, 11, 1228158. doi.org/10.3389/feart.2023.1228158
Aschauer, J., Michel, A., Jonas, T., & Marty, C. (2023). An empirical model to calculate snow depth from daily snow water equivalent: SWE2HS 1.0. Geoscientific Model Development, 16(14), 4063-4081. https://doi.org/10.5194/gmd-16-4063-2023
Griessinger, N., Seibert, J., Magnusson, J., and Jonas, T. (2016). Assessing the benefit of snow data assimilation for runoff modeling in Alpine catchments. Hydrol. Earth Syst. Sci. 20, 3895–3905. doi:10.5194/hess-20-3895-2016
Griessinger, N., Schirmer, M., Helbig, N., Winstral, A., Michel, A., and Jonas, T. (2019). Implications of observation-enhanced energy-balance snowmelt simulations for runoff modeling of Alpine catchments. Adv. Water Resour. 133, 12, 103410. doi:10. 1016/j.advwatres.2019.103410.
Helbig, N., van Herwijnen, A., Magnusson, J., and Jonas, T. (2015). Fractional snowcovered area parameterization over complex topography. Hydrol. Earth Syst. Sci. 19, 1339–1351. doi:10.5194/hess-19-1339-2015.
Helbig, N., Schirmer, M., Magnusson, J., Mäder, F., van Herwijnen, A., Quéno, L., et al. (2021b). A seasonal algorithm of the snow-covered area fraction for mountainous terrain. Cryosphere 15, 4607–4624. doi:10.5194/tc-15-4607-2021.
Jonas, T., Marty, C., and Magnusson, J. (2009). Estimating the snow water equivalent from snow depth measurements in the Swiss Alps. J. Hydrology 378 (1-2), 161–167. doi:10.1016/j.jhydrol.2009.09.021.
Jonas, T.,Webster, C.,Mazzotti, G., and Malle, J. (2020). HPEval: A canopy shortwave radiation transmission model using high-resolution hemispherical images. Agric. For. Meteorology 284, 107903. doi:10.1016/j.agrformet.2020.107903.
Magnusson, J., Gustafsson, D., Husler, F., and Jonas, T. (2014). Assimilation of point SWE data into a distributed snow cover model comparing two contrasting methods. Water Resour. Res. 50, 7816–7835. doi:10.1002/2014WR015302.
Magnusson, J., Wever, N., Essery, R., Helbig, N., Winstral, A., and Jonas, T. (2015). Evaluating snow models with varying process representations for hydrological applications. Water Resour. Res. 51, 2707–2723. doi:10.1002/2014WR016498.
Mazzotti, G., Essery, R. L. H., Webster, C., Malle, J., and Jonas, T. (2020b). Processlevel evaluation of a hyper-resolution forest snow model using distributed multi-sensor observations. Water Resour. Res. 56 (9), e2020WR027572. doi:10.1029/2020WR027572
Mazzotti, G., Webster, C., Essery, R., and Jonas, T. (2021). Increasing the physical representation of forest-snow processes in coarse-resolution models: Lessons learned from upscaling hyper-resolution simulations. Water Resour. Res. 57 (5), e2020WR029064. doi:10.1029/2020WR029064.
Webster, C., Mazzotti, G., Essery, R. L. H., and Jonas, T. (2020). Enhancing airborne LiDAR data for improved forest structure representation in shortwave transmission models. Remote Sens. Environ. 249, 112017. doi:10.1016/j.rse.2020.112017
Webster, C., Essery, R., Mazzotti, G., and Jonas, T. (2023). Using just a canopy height model to obtain lidar-level accuracy in 3D forest canopy shortwave transmissivity estimates. Agric. For. Meteorology 338, 109429. doi:10.1016/j.agrformet.2023.109429.
Winstral, A., Jonas, T., and Helbig, N. (2017). Statistical downscaling of gridded wind speed data using local topography. J. Hydrometeorol. 18 (2), 335–348. doi:10.1175/jhmd-16-0054.1
Winstral, A., Magnusson, J., Schirmer, M., and Jonas, T. (2019). The bias-detecting ensemble: A new and efficient technique for dynamically incorporating observations into physics-based, multilayer snow models. Water Resour. Res. 55, 613–631. doi:10.1029/2018WR024521