Automatic detection of avalanches from optical remote sensed data
2020 - 2024
FinancingComprehensive satellite data, for smaller areas also drone images, with high resolution are well suited for the documentation of avalanche periods. For a (near-) realtime use the SLF is working on automating the mapping.
Background ¶
Information on the spatial distribution and size of avalanches are crucial for avalanche warning, avalanche documentation, mitigation measure design and hazard zonation. Despite this relevance, this information is incomplete today and only available for limited regions and limited intervals. Avalanche mapping from satellite imagery has recently attempted to fill this gap with promising results.
In both, January 2018 and 2019, periods with very high avalanche danger (level 5) occurred over large parts of Switzerland for the first time since 1999. We carried out a comprehensive documentation of those events (Bühler et al., 2019) in cooperation with the BAFU, the cantons Valais and Grisons as well as swisstopo, relying on optical satellite imagery (SPOT 6, 1.5m resolution). During the large-scale mapping over the vast area of ~22’000 km2 (2018: ~12’500 km2; 2019: ~9’500 km2) a total of 24 778 (2018: 18 737; 2019: 6 041) single avalanche polygons were manually mapped (Fig. 1). Additionally, weather independent radar data (TerraSAR-x & Sentinel-1) were tested in some overlapping areas (Leinss et al., 2020).
Reliability of the mappings ¶
To investigate the reliability of the mapped avalanches an evaluation of performance and completeness for both years was conducted in a test area of 180 km2 around Davos, Switzerland. With an overall Probability of Detection (POD) of 0.74 and a Positive Predictive Value (PPV) of 0.88 for avalanches larger than size 1, SPOT reliably mapped the majority of avalanches. For the same area Sentinel-1 (radar) mapped the avalanches with a POD of 0.27 and a PPV of 0.87 (avalanches ≥ size 2). These differences stem from differing detection rates per avalanche size shown in Figure 3. Both methods show declining ability to comprehensively map avalanches with decreasing size, for Sentinel-1 this decline is however more pronounced. With a PPV of 0.81 the mapping from Sentinel-2 (optical, less resolution than SPOT) would be reliable but the POD of 0.06 shows that very few avalanches are mapped altogether.
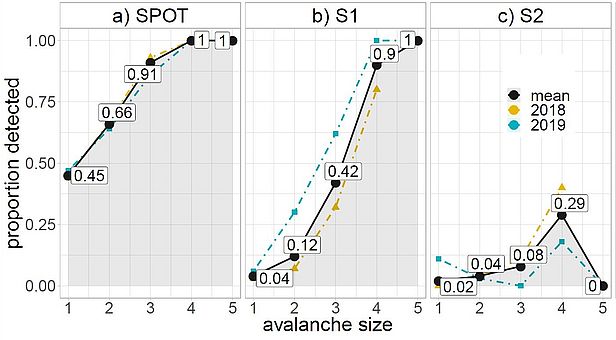
With these investigations we, for the first time, investigated the reliability and accuracy of avalanche mappings from different satellite sensors which may cover large regions. Summarizing, our analysis showed that the SPOT mapping holds great potential for comprehensive mapping of avalanches whereas the Sentinel-1 mapping is quite reliable for larger avalanches (size 3 to 5). The performance of the mapping with Sentinel-2, on the other hand, was not satisfactory (Hafner et al., 2020).
Automation of the mapping with machine learning (ML) ¶
For the manual outline digitization from SPOT, including attribute recording, we found that an average of two minutes is required per avalanche. This leads to a significant total mapping time and therefore high costs once large areas with numerous avalanches are considered. In order to speed up this process we work on an automation of the mapping relying on machine learning in close collaboration with the EcoVision Lab at ETH Zurich. Relying on the datasets from 2018 and 2019 for training, evaluation and testing we trained a DeepLabV3+. DeepLabV3+ is a popular, fully convolutional semantic segmentation model that has been used successfully with a variety of datasets. We slightly adapted the model to explicitly incorporate the terrain, which is essential for avalanches.
Our validation has shown that the model is as good as human experts at identifying avalanches in SPOT6/7 imagery. For future applications we will investigate how well the model works in differing illumination and snow conditions as well as with data from other sensors.
Project AvalMapper ¶
For AvalMapper we collaborate with the Autonomous System Lab (ASL) at ETH Zurich pairing their know-how in developing fixed-wing UAVs for specific applications with our experience with drones in high alpine terrain and avalanche mapping from remote sensed imagery. Together we work on developing a drone autonomously documenting every avalanche period in the region of Davos with a subsequent automated mapping of avalanches from acquired imagery. For this purpose, we will first test drones and cameras to meet our requirements, while simultaneously refining our algorithm for automating the avalanche mapping for very high resolution (VHR) optical drone data.
Video 1: Impressions from the AVALMAPPER testflights in winter 2021/22.